Overview of the Open Images Challenge
The challenge is based on the Open Images dataset. The images of the dataset are very diverse and often contain complex scenes with several objects (explore the dataset). The 2019 edition of the challenge had three tracks:
- Object Detection: predicting a tight bounding box around all object instances of 500 classes.
- Visual Relationship Detection: detecting pairs of objects in particular relations.
- Instance Segmentation: predicting the outlines of object instances from 300 classes.
We hope that having a single dataset with unified annotations for image classification, object detection, visual relationship detection, and instance segmentation will hopefully promote studying these tasks jointly and stimulate progress towards genuine scene understanding.
The 2018 and 2019 editions of the challenge were run by Google AI in partnership with Kaggle. The results are available here:
The evaluation servers of the 2019 challenge are still accessible. This enables evaluating new methods on the hidden challenge dataset and to compare properly to previous results. Please check the Train data and Evaluation section.
In 2020, Google AI will not run a separate edition of Open Images Challenge. However, the Object Detection and Instance Segmentation tracks are included into the Robust Vision Challenge 2020.
Robust Vision Challenge 2020
The Object Detection and Instance Segmentation tracks are included into Robust Vision Challenge 2020!
The goal of the Robust Vision Challenge is to foster the development of vision systems that are robust and consequently perform well on a variety of datasets with different characteristics. The challenge features seven diverse compute vision tasks. The performance for each task is measured across from 3 to 8 challenging benchmarks with different characteristics, e.g., indoors vs. outdoors, real vs. synthetic, sunny vs. bad weather, different sensors. The final ranking in the challenge within each task will be determined using Schulze Proportional Ranking (PR) method. More information about the challenge visit Robust Vision Challenge webite.
For the Robust Vision Challenge, the development kit v1.0 is released. It supports downloading of relevant Open Images groundtruth files as well as conversion to the unified RVC format. The data and metrics for OID benchmark are described in the next section.
Evaluation servers on Kaggle are accepting submission:
Stay tuned for the submission instructions for Robust Vision Challenge 2020.
Train data and Evaluation
The challenge set used to report results can be downloaded from Kaggle. Training and validation sets can be downloaded from Challenge Download section. The evaluation protocols for each track are described in the Evaluation section. The python implementation of all three evaluation protocols is released as a part of the Tensorflow Object Detection API. The evaluation servers of the Open Images Challenge accept submissions:
To create a submission, click on 'Late Submissions' / 'Submit Predictions' , accept terms and conditions and upload a solution file. Note that when you are submitting to RVC servers your score will be visible on the public leaderboard and you will only be able to see the private score after the challenge ends. For VRD evaluation server, both Public and Private scores will be available for you but not visible on the public leaderboard.
Object detection track
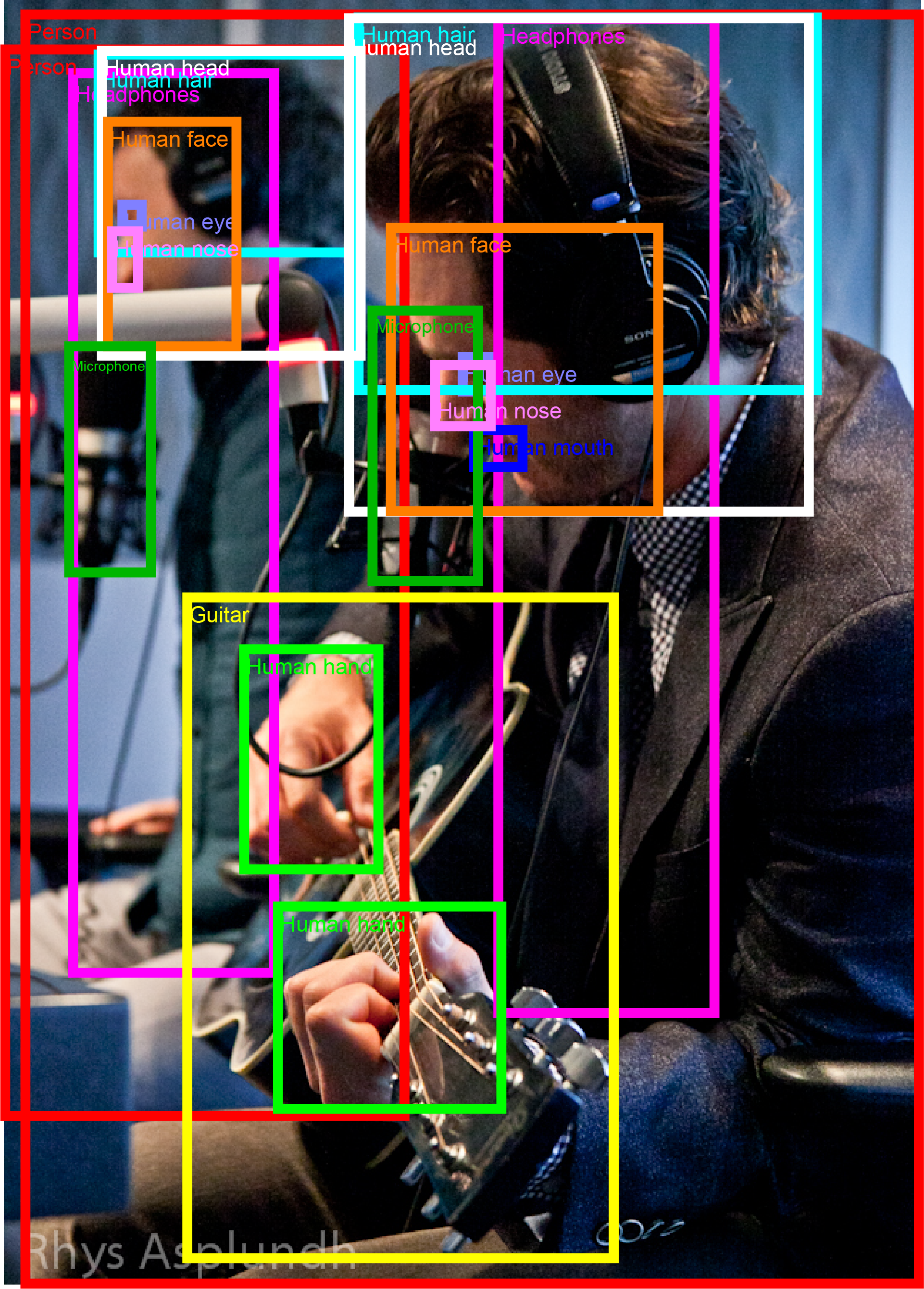

The Object Detection track covers 500 classes out of the 600 annotated with bounding boxes in Open Images V5 (see Table 1 for the details). The evaluation metric is mean Average Precision (mAP) over the 500 classes, see details here.
Table 1: Object Detection track annotations on train and validation set.
Image-Level Labels | Bounding boxes | |
---|---|---|
Train | 5,743,460 | 12,195,144 |
Validation | 193,300 | 226,811 |
Visual relationship detection track
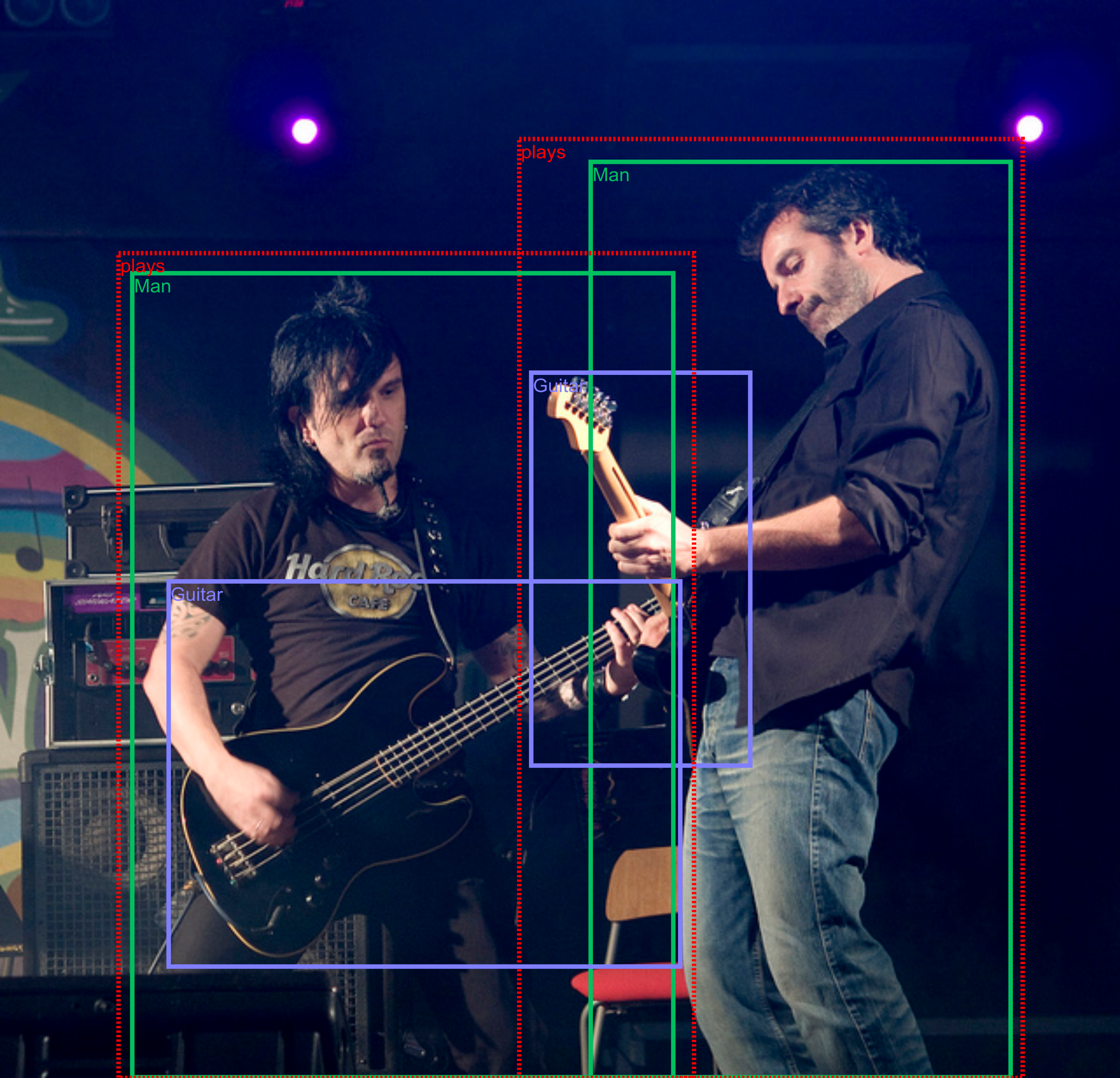
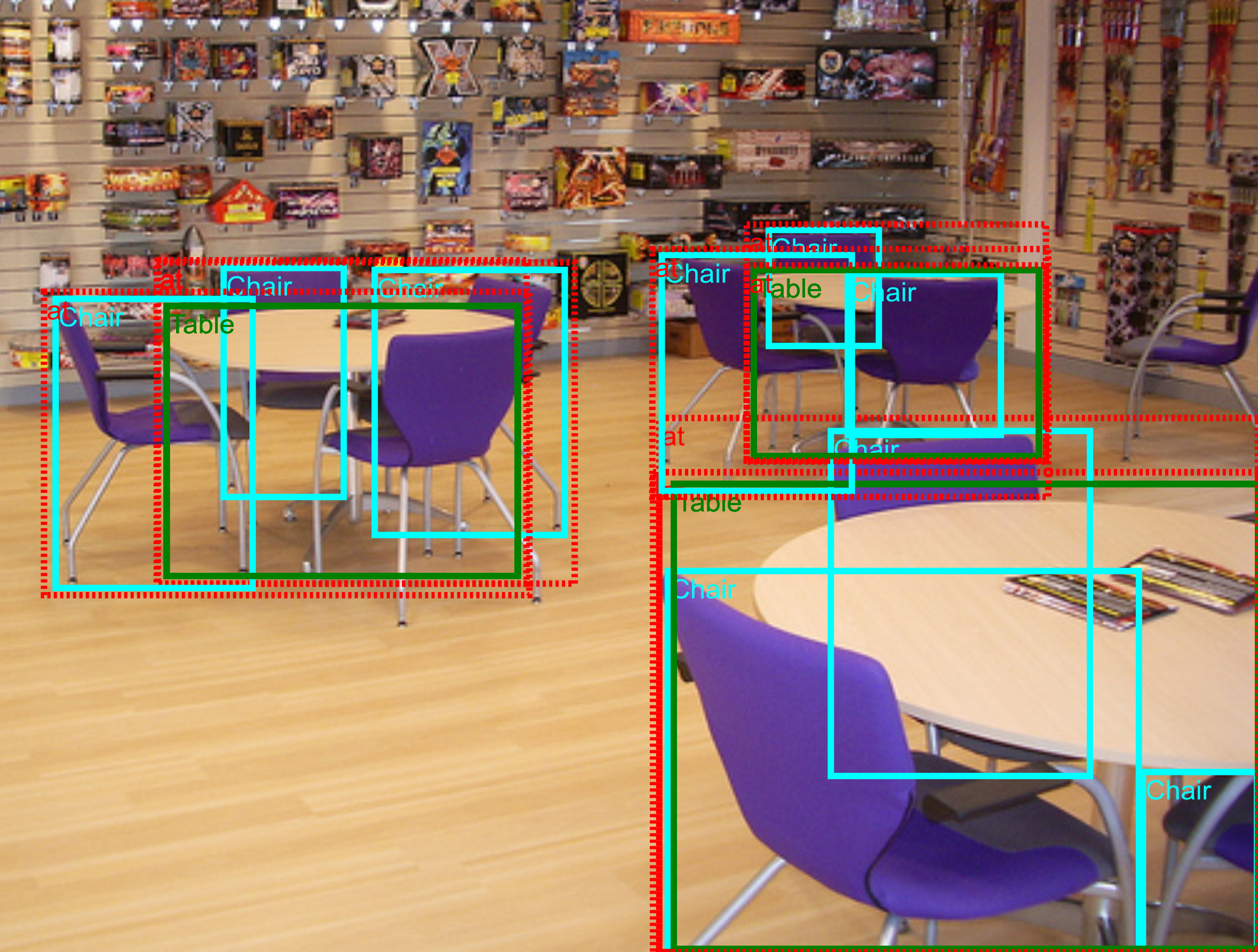
The Visual Relationships Detection track requires detecting relationships connecting two objects. These include both human-object relationships and object-object relationships, as well as object-attribute relationships (more information in Table 2). For evaluation a linear combination of mean Average Precision (mAP) and retrieval metrics on phrase detection and relationship detection tasks is used (see details here).
Table 2: Visual Relationships Detection metadata information.
Classes | Relationships | Visual attributes | Distinct relationship triplets | |
---|---|---|---|---|
Relationships connecting two objects | 57 | 9 | - | 287 |
Relationship "is" (visual attributes) | 23 | 1 | 5 | 42 |
Total | 57 | 10 | 5 | 329 |
Instance segmentation track
The instance segmentation track is new for the 2019 edition of the Challenge. This track covers 300 classes out of Open Images V5 (see Table 3 for the details). The evaluation metric computes mean AP (mAP) using mask-to-mask matching over the 300 classes. The evaluation metric is described in detail here.
Table 3: Instance segmentation track annotations on train and validation sets.
Image-Level Labels | Bounding boxes | Masks | |
---|---|---|---|
Train | 2,987,501 | 5,984,294 | 2,125,530 |
Validation | 84,348 | 101,943 | 23,366 |
Contacts
For challenge-related questions please contact oid-challenge-contact. To receive news about the challenge and the Open Images dataset, subscribe to Open Images newsletter here.